Part 1: Social Media Data
Workshop: Social Media, Data Analysis, & Cartograpy, WS 2024/25
Alexander Dunkel
Leibniz Institute of Ecological Urban and Regional Development,
Transformative Capacities & Research Data Centre & Technische Universität Dresden,
Institute of Cartography
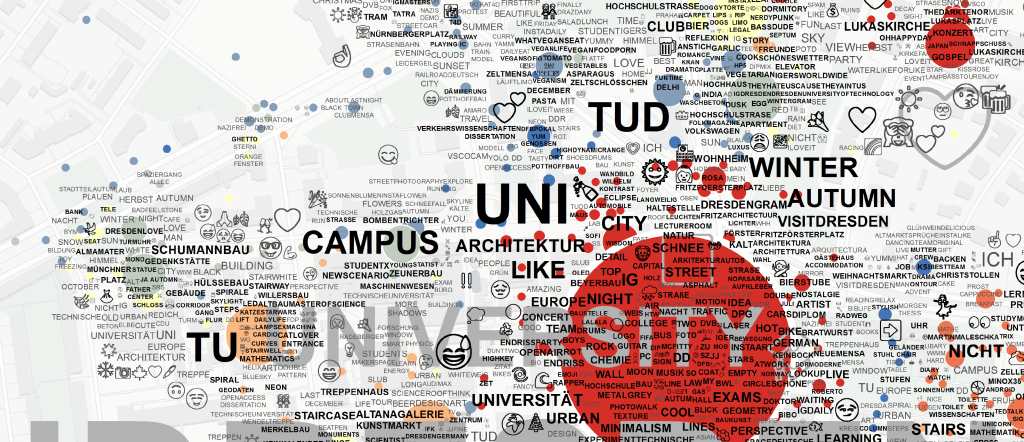
1. Link the workshop environment centrally from the project folder at ZIH:
Select the cell below and click CTRL+ENTER to run the cell. Once the *
(left of the cell) turns into a number (1
), the process is finished.
!cd .. && sh activate_workshop_envs.sh
Refresh the browser window afterwards with F5 and select 01_intro_env
in the top-right corner. Wait until the [*]
disappears.
Refresh the browser window afterwards with F5, so that the environment becomes available on the top-right dropdown list of kernels.
Well done!
Welcome to the IfK Social Media, Data Science, & Cartograpy workshop.This is the first notebook in a series of four notebooks:
- Introduction to Social Media data, jupyter and python spatial visualizations
- Introduction to privacy issues with Social Media data and possible solutions for cartographers
- Specific visualization techniques example: TagMaps clustering
- Specific data analysis: Topic Classification
Open these notebooks through the file explorer on the left side.
- Please make sure that "01_intro_env" is shown on the top-right corner. If not, click & select.
- If the "01_intro_env" is not listed, save the notebook (CTRL+S), and check the list again after a few seconds
- use SHIFT+ENTER to walk through cells in the notebook
User Input
- We'll highlight sections where you can change parameters
- All other code is intended to be used as-is, do not change
FAQ
If you haven't worked with jupyter, these are some tips:
- Jupyter Lab allows to interactively execute and write annotated code
- There are two types of cells: Markdown cells contain only text (annotations), Code cells contain only python code
- Cells can be executed by SHIFT+Enter
- The output will appear below
- States of python will be kept in-between code cells: This means that a value assigned to a variable in one cell remains available afterwards
- This is accomplished with IPython, an interactive version of python
- Important: The order in which cells are executed does not have to be linear. It is possible to execute any cell in any order. Any code in the cell will use the current "state" of all other variables. This also allows you to update variables.
LINKS
Some links
- This notebook is prepared to be used from the TUD ZIH Jupyter Hub
- .. but it can also be run locally. For example, with our IfK Jupyter Lab Docker Container
- The contents of this workshop are available in a git repository
- There, you'll also find static HTML versions of these notebooks.
AVAILABLE PACKAGES
This python environment is prepared for spatial data processing/ cartography.
The following is a list of the most important packages, with references to documentation:
- Geoviews
- Holoviews
- Bokeh
- hvPlot
- Geopandas
- Pandas
- Numpy
- Matplotlib
- Contextily
- Colorcet
- Cartopy
- Shapely
- Pyproj
- pyepsg
- Mapclassify
- Seaborn
- Xarray
- Tagmaps
- lbsnstructure
If you want to run these notebooks at home, try the IfK Jupyter Docker Container, which includes the same packages.
Preparations¶
We are creating several output graphics and temporary files.
These will be stored in the subfolder notebooks/out/.
from pathlib import Path
OUTPUT = Path.cwd() / "out"
OUTPUT.mkdir(exist_ok=True)
Syntax: pathlib.Path() / "out" ?
Python pathlib provides a convenient, OS independend access to local filesystems. These paths work independently of the OS used (e.g. Windows or Linux). Path.cwd() gets the current directory, where the notebook is running. See the docs..To reduce the code shown in this notebook, some helper methods are made available in a separate file.
Load helper module from ../py/module/tools.py
.
import sys
module_path = str(Path.cwd().parents[0] / "py")
if module_path not in sys.path:
sys.path.append(module_path)
from modules import tools
Activate autoreload of changed python files:
%load_ext autoreload
%autoreload 2
Introduction: VGI and Social Media Data¶
Broadly speaking, GI and User Generated Content can be classified in the following three categories of data:
- Authoritative data that follows objective criteria of measurement such as Remote Sensing, Land-Use, Soil data etc.
- Explicitly volunteered data, such as OpenStreetMap or Wikipedia. This is typically collected by many people, who collaboratively work on a common goal and follow more or less specific contribution guidelines.
- Subjective information sources
- Explicit: e.g. Surveys, Opinions etc.
- Implicit: e.g. Social Media
Social Media data belongs to the third category of subjective information, representing certain views held by groups of people. The difference to Surveys is that there is no interaction needed between those who analyze the data and those who share the data
online, e.g. as part of their daily communication.
Social Media data is used in marketing, but it is also increasingly important for understanding people's behaviour, subjective values, and human-environment interaction, e.g. in citizen science and landscape & urban planning.
In this notebook, we will explore basic routines how Social Media and VGI can be accessed through APIs and visualized in python.
Social Media APIs¶
- Social Media data can be accessed through public APIs.
- This will typically only include data that is explicitly made public by users.
- Social Media APIs exist for most networks, e.g. Flickr, Twitter, or Instagram
Privacy?
We'll discuss legal, ethical and privacy issues with Social Media data in the second notebook: 02_hll_intro.ipynbInstagram Example¶
- Retrieving data from APIs requires a specific syntax that is different for each service.
- commonly, there is an endpoint (a url) that returns data in a structured format (e.g. json)
- most APIs require you to authenticate, but not all (e.g. Instagram, commons.wikimedia.org)
But the Instagram API was discontinued!
- Instagram discontinued their official API in October 2018. However, their Web-API is still available, and can be accessed even without authentication.
- One rationale is that users not signed in to Instagram can have "a peek" at images, which provides significant attraction to join the network.
- We'll discuss questions of privacy and ethics in the second notebook.
Load Instagram data for a specific Location ID. Get the location ID from a search on Instagram first.
location_id = "1893214" # "Großer Garten" Location
query_url = f'https://i.instagram.com/api/v1/locations/web_info/?location_id={location_id}&show_nearby=false'
Use your own location
Optionally replace "1893214" with another location above. You can search on instagram.com and extract location IDs from the URL. Examples:657492374396619
Beutlerpark, Dresden270772352
Knäbäckshusens beach, Sweden- You name it
Syntax: f'{}' ?
This is called an f-string, a convenient python convention to concat strings and variables.Use your own json, in case automatic download did not work.
- Since it is likely that access without login will not be possible for all in the workshop, we have provided a sample json, that will be retrieved if no access is possible
- If automatic download does not work below, the code will automatically retrieve the archive json
- you can use your own json below, by saving a (e.g. park.json) and moving it, via drag-and-drop, to the out folder on the left.
First, try to get the json-data without login. This may or may not work:
import requests
json_text = None
response = requests.get(
url=query_url, headers=tools.HEADER)
if response.status_code == 429 \
or "/login/" in response.url \
or '"status":"fail"' in response.text \
or '<!DOCTYPE html>' in response.text:
print(f"Loading live json failed: {response.text[:250]}")
else:
json_text = response.text
# write to temporary file
with open(OUTPUT / f"live_{location_id}.json", 'w') as f:
f.write(json_text)
print("Loaded live json")
"Loaded live json"? Successful
our/sample.json
. If such a file exists, it will be loaded:if not json_text:
# check if manual json exists
local_json = [json for json in OUTPUT.glob('*.json')]
if len(local_json) > 0:
# read local json
with open(local_json[0], 'r') as f:
json_text = f.read()
print("Loaded local json")
Syntax: [x for x in y] ?
This is called a list comprehension, a convenient python convention to to create lists (from e.g. generators etc.).Otherwise, if neither live nor local json has been loaded, load sample json from archive:
if not json_text:
sample_url = tools.get_sample_url()
sample_json_url = f'{sample_url}/download?path=%2F&files=1893214.json'
response = requests.get(url=sample_json_url)
json_text = response.text
print("Loaded sample json")
Turn text into json format:
import json
json_data = json.loads(json_text)
Have a peek at the returned data.
We can use json.dumps
for this and limit the output to the first 550 characters [0:550]
.
print(json.dumps(json_data, indent=2)[0:550])
The json data is nested. Values can be accessed with dictionary keys.
from IPython.core.display import HTML
total_cnt = json_data["native_location_data"]["location_info"].get("media_count")
display(HTML(
f'''<details><summary>Working with the JSON Format</summary>
The json data is nested. Values can be accessed with dictionary keys. <br>For example,
for the location <strong>{location_id}</strong>,
the total count of available images on Instagram is <strong>{total_cnt:,.0f}</strong>.
</details>
'''))
You can find the media under:
for ix in range(1, 5):
display(str(json_data["native_location_data"]["ranked"]["sections"][ix]["layout_content"]["medias"][0])[0:100])
Where [ix]
is a pointer to a list of three media per row. Below, we loop through these lists and combine them to a single dataframe.
Dataframes are a flexible data analytics interface that is available with pandas.DataFrame()
. Most tabular data can be turned into a dataframe.
Dataframe ?
A pandas dataframe is the typical tabular data format used in python data science. Most data can be directly converted to a DataFrame.import pandas as pd
df = None
# loop through media, skip first item
for data in json_data["native_location_data"]["ranked"]["sections"][1:]:
df_new = pd.json_normalize(
data, errors="ignore")
if df is None:
df = df_new
else:
df = pd.concat([df, df_new])
Display
df.transpose()
See an overview of all columns/attributes available at this json level:
from IPython.core.display import HTML
display(HTML(f"<details><summary>Click</summary><code>{[col for col in df.columns]}</code></summary>"))
df['layout_content.medias'].iloc[0]
.
We want to extract URLs of images, so that we can download images in python and display inside the notebook.
First, extract URLs of images:
url_list = []
for media_grid in df['layout_content.medias']:
for media_row in media_grid:
for media in media_row["media"]["image_versions2"]["candidates"]:
url_list_new = []
url = media["url"]
url_list_new.append(url)
url_list.extend(url_list_new)
View the first few (15) images
First, define a function.
PIL Library
resize
function
and the ImageFilter.BLUR
filter. The Image is processed in-memory.
Afterwards, plt.subplot()
is used to plot images in a row. Can you modify the code to plot images
in a multi-line grid?
from typing import List
import matplotlib.pyplot as plt
from PIL import Image, ImageFilter
from io import BytesIO
def image_grid_fromurl(url_list: List[str]):
"""Load and show images in a grid from a list of urls"""
count = len(url_list)
plt.figure(figsize=(11, 18))
for ix, url in enumerate(url_list[:15]):
r = requests.get(url=url)
i = Image.open(BytesIO(r.content))
resize = (150, 150)
i = i.resize(resize)
i = i.filter(ImageFilter.BLUR)
plt.subplots_adjust(bottom=0.3, right=0.8, top=0.5)
ax = plt.subplot(3, 5, ix + 1)
ax.axis('off')
plt.imshow(i)
Use the function to display images from "node.display_url" column.
All images are public and available without Instagram login, but we still blur images a bit, as a precaution and a measure of privacy.
image_grid_fromurl(
url_list)
Get Images for a hashtag
For example, the location-feed for the Großer Garten is available at: https://www.instagram.com/explore/hashtag/park/.
Creating Maps¶
- Frequently, VGI and Social Media data contains references to locations such as places or coordinates.
- Most often, spatial references will be available as latitude and logitude (decimal degrees and WGS1984 projection).
- To demonstrate integration of data, we are now going to query another API, commons.wikimedia.com, to get a list of places near certain coordinates.
Choose a coordinate
- Below, coordinates for the Großer Garten are used. They can be found in the json link.
- Substitute with your own coordinates of a chosen place.
lat = 51.03711
lng = 13.76318
Get list of nearby places using commons.wikimedia.org's API:
query_url = f'https://commons.wikimedia.org/w/api.php'
params = {
"action":"query",
"list":"geosearch",
"gsprimary":"all",
"gsnamespace":14,
"gslimit":50,
"gsradius":1000,
"gscoord":f'{lat}|{lng}',
"format":"json"
}
response = requests.get(
url=query_url, params=params)
if response.status_code == 200:
print(f"Query successful. Query url: {response.url}")
json_data = json.loads(response.text)
print(json.dumps(json_data, indent=2)[0:500])
Get List of places.
location_dict = json_data["query"]["geosearch"]
Turn into DataFrame.
df = pd.DataFrame(location_dict)
display(df.head())
df.shape
If we have queried 50 records, we have reached the limit specified in our query. There is likely more available, which would need to be queried using subsequent queries (e.g. by grid/bounding box). However, for the workshop, 50 locations are enough.
Modify data.: Replace "Category:" in column title.
- Functions can be easily applied to subsets of records in DataFrames.
- although it is tempting, do not iterate through records
- dataframe vector-functions are almost always faster and more pythonic
df["title"] = df["title"].str.replace("Category:", "")
df.rename(
columns={"title":"name"},
inplace=True)
Turn DataFrame into a GeoDataFrame
GeoDataframe ?
A geopandas GeoDataFrame is the spatial equivalent of a pandas dataframe. It supports all operations of DataFrames, plus spatial operations. A GeoDataFrame can be compared to a Shapefile in (e.g.), QGis.import geopandas as gp
gdf = gp.GeoDataFrame(
df, geometry=gp.points_from_xy(df.lon, df.lat))
Set projection, reproject
Projections in Python
- Most available spatial packages have more or less agreed on a standard format for handling projections in python.
- The recommended way is to define projections using their epsg ids, which can be found using epsg.io
- Note that, sometimes, the projection-string refers to other providers, e.g. for Mollweide, it is "ESRI:54009"
CRS_PROJ = "epsg:3857" # Web Mercator
CRS_WGS = "epsg:4326" # WGS1984
gdf.crs = CRS_WGS # Set projection
gdf = gdf.to_crs(CRS_PROJ) # Project
gdf.head()
Display location on a map
- Maplotlib and contextily provide one way to plot static maps.
- we're going to show another, interactive map renderer afterwards
Import contextily, which provides static background tiles to be used in matplot-renderer.
import contextily as cx
1. Create a bounding box for the map
x = gdf.loc[0].geometry.x
y = gdf.loc[0].geometry.y
margin = 1000 # meters
bbox_bottomleft = (x - margin, y - margin)
bbox_topright = (x + margin, y + margin)
gdf.loc[0] ?
gdf.loc[0]
is the loc-indexer from pandas. It means: access the first record of the (Geo)DataFrame..geometry.x
is used to access the (projected) x coordinate geometry (point). This is only available for GeoDataFrame (geopandas)
2. Create point layer, annotate and plot.
- With matplotlib, it is possible to adjust almost every pixel individual.
- However, the more fine-tuning is needed, the more complex the plotting code will get.
- In this case, it is better to define methods and functions, to structure and reuse code.
Code complexity
from matplotlib.patches import ArrowStyle
# create the point-layer
ax = gdf.plot(
figsize=(10, 15),
alpha=0.5,
edgecolor="black",
facecolor="red",
markersize=300)
# set display x and y limit
ax.set_xlim(
bbox_bottomleft[0], bbox_topright[0])
ax.set_ylim(
bbox_bottomleft[1], bbox_topright[1])
# turn of axes display
ax.set_axis_off()
# add callouts
# for the name of the places
for index, row in gdf.iterrows():
# offset labels by odd/even
label_offset_x = 30
if (index % 2) == 0:
label_offset_x = -100
label_offset_y = -30
if (index % 4) == 0:
label_offset_y = 100
ax.annotate(
text=row["name"].replace(' ', '\n'),
xy=(row["geometry"].x, row["geometry"].y),
xytext=(label_offset_x, label_offset_y),
textcoords="offset points",
fontsize=8,
bbox=dict(
boxstyle='round,pad=0.5',
fc='white',
alpha=0.5),
arrowprops=dict(
mutation_scale=4,
arrowstyle=ArrowStyle(
"simple, head_length=2, head_width=2, tail_width=.2"),
connectionstyle=f'arc3,rad=-0.3',
color='black',
alpha=0.2))
cx.add_basemap(
ax, alpha=0.5,
source=cx.providers.OpenStreetMap.Mapnik)
There is space for some improvements:
- Labels overlap. There is a package callec adjust_text that allows to reduce overlapping annotations in mpl automatically. This will take more time, however.
- Line breaks after short words don't look good. Use the native
textwrap
function.
Code execution time
from adjustText import adjust_text
import textwrap
# create the point-layer
ax = gdf.plot(
figsize=(15, 25),
alpha=0.5,
edgecolor="black",
facecolor="red",
markersize=300)
# set display x and y limit
ax.set_xlim(
bbox_bottomleft[0], bbox_topright[0])
ax.set_ylim(
bbox_bottomleft[1], bbox_topright[1])
# turn of axes display
ax.set_axis_off()
# add callouts
# for the name of the places
texts = []
for index, row in gdf.iterrows():
texts.append(
plt.text(
s='\n'.join(textwrap.wrap(
row["name"], 18, break_long_words=True)),
x=row["geometry"].x,
y=row["geometry"].y,
horizontalalignment='center',
fontsize=8,
bbox=dict(
boxstyle='round,pad=0.5',
fc='white',
alpha=0.5)))
adjust_text(
texts, autoalign='y', ax=ax,
arrowprops=dict(
arrowstyle="simple, head_length=2, head_width=2, tail_width=.2",
color='black', lw=0.5, alpha=0.2, mutation_scale=4,
connectionstyle=f'arc3,rad=-0.3'))
cx.add_basemap(
ax, alpha=0.5,
source=cx.providers.OpenStreetMap.Mapnik)
Further improvements
- Try adding a title. Suggestion: Use the explicit
ax
object. - Add a scale bar. Suggestion: Use the pre-installed package
matplotlib-scalebar
- Change the basemap to Aerial.
Have a look at the available basemaps:
cx.providers.keys()
And a look at the basemaps for a specific provider:
cx.providers.CartoDB.keys()
Interactive Maps¶
Plot with Holoviews/ Geoviews (Bokeh)
import holoviews as hv
import geoviews as gv
from cartopy import crs as ccrs
hv.notebook_extension('bokeh')
Create point layer:
places_layer = gv.Points(
df,
kdims=['lon', 'lat'],
vdims=['name', 'pageid'],
label='Place')
Make an additional query, to request pictures shown in the area from commons.wikimedia.org
query_url = f'https://commons.wikimedia.org/w/api.php'
params = {
"action":"query",
"list":"geosearch",
"gsprimary":"all",
"gsnamespace":6,
"gsradius":1000,
"gslimit":500,
"gscoord":f'{lat}|{lng}',
"format":"json"
}
response = requests.get(
url=query_url, params=params)
print(response.url)
json_data = json.loads(response.text)
df_images = pd.DataFrame(json_data["query"]["geosearch"])
df_images.head()
- Unfortunately, this didn't return any information for the pictures. We want to query the thumbnail-url, to show this on our map.
- For this, we'll first set the pageid as the index (=the key),
- and we use this key to update our Dataframe with thumbnail-urls, retrievd from an additional API call
Set Column-type as integer:
df_images["pageid"] = df_images["pageid"].astype(int)
Set the index to pageid:
df_images.set_index("pageid", inplace=True)
df_images.head()
Load additional data from API: Place Image URLs
params = {
"action":"query",
"prop":"imageinfo",
"iiprop":"timestamp|user|userid|comment|canonicaltitle|url",
"iiurlwidth":200,
"format":"json"
}
See the full list of available attributes.
Query the API for a random sample of 50 images:
%%time
from IPython.display import clear_output
from datetime import datetime
count = 0
df_images["userid"] = 0 # set default value
for pageid, row in df_images.sample(n=min(50, len(df_images))).iterrows():
params["pageids"] = pageid
try:
response = requests.get(
url=query_url, params=params)
except OSError:
print(
"Connection error: Either try again or "
"continue with limited number of items.")
break
json_data = json.loads(response.text)
image_json = json_data["query"]["pages"][str(pageid)]
if not image_json:
continue
image_info = image_json.get("imageinfo")
if image_info:
thumb_url = image_info[0].get("thumburl")
count += 1
df_images.loc[pageid, "thumb_url"] = thumb_url
clear_output(wait=True)
display(HTML(
f"Queried {count} image urls, "
f"<a href='{response.url}'>last query-url</a>."))
# assign additional attributes
df_images.loc[pageid, "user"] = image_info[0].get("user")
df_images.loc[pageid, "userid"] = image_info[0].get("userid")
timestamp = pd.to_datetime(image_info[0].get("timestamp"))
df_images.loc[pageid, "timestamp"] = timestamp
df_images.loc[pageid, "title"] = image_json.get("title")
Connection error
?
The Jupyter Hub @ ZIH is behind a proxy. Sometimes, connections will get reset.
In this case, either execute the cell again or continue with the limited number of items
retrieved so far.
%%time
?
IPython has a number of built-in "magics",
and %%time
is one of them. It will output the total execution time of a cell.
- We have only queried 50 of our 100 images for urls.
- To view only the subset of records with urls, use boolean indexing
df_images[
df_images["userid"] != 0].head()
- What happens here in the background is that
df_images["userid"] != 0
returns True for all records where "iserid" is not 0 (the default value). - In the second step, this is used to
slice
records using the boolean indexing:df_images[Condition=True]
Next (optional) step: Save queried data to CSV
- dataframes can be easily saved (and loaded) to (from) CSV using pd.DataFrame.to_csv()
- there's also pd.DataFrame.to_pickle()
- a general recommendation is to use
to_csv()
for archive purposes .. - ..and
to_pickle()
for intermediate, temporary files stored and loaded to/from disk
df_images[df_images["userid"] != 0].to_csv(
OUTPUT / "wikimedia_commons_sample.csv")
Open CSV in the Explorer
- Click the link wikimedia_commons_sample.csv, to have a look at the structure of the generated CSV.
- Jupyter Lab provides several renderers for typical file formats such as CSV, JSON, or HTML
- Notice some of the Full User Names provides in the list. We will use this sample data in the second notebook, to explore privacy aspects of VGI.
Create two point layers, one for images with url and one for those without:
images_layer_thumbs = gv.Points(
df_images[df_images["thumb_url"].notna()],
kdims=['lon', 'lat'],
vdims=['thumb_url', 'user', 'timestamp', 'title'],
label='Picture (with thumbnail)')
images_layer_nothumbs = gv.Points(
df_images[df_images["thumb_url"].isna()],
kdims=['lon', 'lat'],
label='Picture')
kdims and vdims?
- kdims refers to the key-dimensions, which provide the primary references for plotting to x/y axes (coordinates)
- vdims refers to the value-dimensions, which provide additional information that is shown in the plot (e.g. colors, size, tooltips)
- Each string in the list refers to a column in the dataframe.
- Anything that is not included here in the layer-creation cannot be shown during plotting.
margin = 500 # meters
bbox_bottomleft = (x - margin, y - margin)
bbox_topright = (x + margin, y + margin)
from bokeh.models import HoverTool
from typing import Dict, Optional
def get_custom_tooltips(
items: Dict[str, str], thumbs_col: Optional[str] = None) -> str:
"""Compile HoverTool tooltip formatting with items to show on hover
including showing a thumbail image from a url"""
tooltips = ""
if items:
tooltips = "".join(
f'<div><span style="font-size: 12px;">'
f'<span style="color: #82C3EA;">{item}:</span> '
f'@{item}'
f'</span></div>' for item in items)
tooltips += f'''
<div><img src="@{thumbs_col}" alt="" style="height:170px"></img></div>
'''
return tooltips
Bokeh custom styling
- The above code to customize Hover tooltips is shown for demonstration purposes only
- As it is obvious, such customization can become quite complex
- Below, it is also shown how to use the default Hover tooltips, which is the recommended way for most situations
- In this case, Holoviews will display tooltips for any DataFrame columns that are provided as vdims (e.g.: vdims=['thumb_url', 'user', 'timestamp', 'title'])
def set_active_tool(plot, element):
"""Enable wheel_zoom in bokeh plot by default"""
plot.state.toolbar.active_scroll = plot.state.tools[0]
# prepare custom HoverTool
tooltips = get_custom_tooltips(
thumbs_col='thumb_url', items=['title', 'user', 'timestamp'])
hover = HoverTool(tooltips=tooltips)
gv_layers = hv.Overlay(
gv.tile_sources.EsriImagery * \
places_layer.opts(
tools=['hover'],
size=20,
line_color='black',
line_width=0.1,
fill_alpha=0.8,
fill_color='red') * \
images_layer_nothumbs.opts(
size=5,
line_color='black',
line_width=0.1,
fill_alpha=0.8,
fill_color='lightblue') * \
images_layer_thumbs.opts(
size=10,
line_color='black',
line_width=0.1,
fill_alpha=0.8,
fill_color='lightgreen',
tools=[hover])
)
Combining Layers
- The syntax to combine layers is either * or +
- * (multiplay) will overlay layers
- + (plus) will place layers next to each other, in separate plots
- The \ (backslash) is python's convention for line continuation,
to break long lines - The resulting layer-list is a hv.Overlay, which can be used for defining global plotting criteria
Display map
layer_options = {
"projection":ccrs.GOOGLE_MERCATOR,
"title":df.loc[0, "name"],
"responsive":True,
"xlim":(bbox_bottomleft[0], bbox_topright[0]),
"ylim":(bbox_bottomleft[1], bbox_topright[1]),
"data_aspect":0.45, # maintain fixed aspect ratio during responsive resize
"hooks":[set_active_tool]
}
gv_layers.opts(**layer_options)
Store map as static HTML file
Unset data_aspect
and store standalone HTML file that automatically resizes to full height of browser:
layer_options["data_aspect"] = None
hv.save(
gv_layers.opts(**layer_options), OUTPUT / f'geoviews_map.html', backend='bokeh')
Open map in new tab
Create Notebook HTML¶
- For archive purposes, we can convert the entire notebook, including interactive maps and graphics, to an HTML file.
- The command is invoked through the exclamation mark (!), which means: instead of python, use the command line.
Steps:
- Create a single HTML file in ./out/ folder
- disable logging, except errors (
>&- 2>&-
) - use nbconvert template
!jupyter nbconvert --to html_toc \
--output-dir=./out/ ./01_raw_intro.ipynb \
--template=../nbconvert.tpl \
--ExtractOutputPreprocessor.enabled=False >&- 2>&- # create single output file
Summary¶
There are many APIs
- But it is quite complex to query data, since each API has its own syntax
- Each API also returns data structured differently
- Just because it is possible to access data doesn't mean that it is allowed or ethically correct to use the data (e.g. Instagram)
There are (some) Solutions
- Typically, you would not write raw queries using request. For many APIs, packages exist that ease workflows.
- We have prepared LBSN Structure, a common format to handle cross-network Social Media data (e.g. Twitter, Flickr, Instagram). Using this structure reduces the work that is necessary, it also allows visualizations to be adpated to other data easily.
- Privacy is critical, and we will explore one way to reduce the amount of data stored in the follwing notebook.
This is just an introduction
We have only covered a small number of steps. If you want to continue, we recommend trying the following tasks:
- Start and run this notebook locally, on your computer, for example, using our IfK Jupyter Lab Docker Container, or re-create the environment manually with Miniconda
- Explore further visualization techniques, for example:
- Create a static line or scatter plot for temporal contribution of the
df_images
dataframe, see Pandas Visualization - Repeat the same with Holoviews, to create an interactive line/scatter plot.
- Create a static line or scatter plot for temporal contribution of the
Contributions:
- 2021 Workshop: Multigrid display was contributed by Silke Bruns (MA), many thanks!
plt.subplots_adjust(bottom=0.3, right=0.8, top=0.5)
ax = plt.subplot(3, 5, ix + 1)
root_packages = [
'python', 'adjusttext', 'contextily', 'geoviews', 'holoviews', 'ipywidgets',
'ipyleaflet', 'geopandas', 'cartopy', 'matplotlib', 'shapely',
'bokeh', 'fiona', 'pyproj', 'ipython', 'numpy']
tools.package_report(root_packages)